Aditya Chattopadhyay
Ph.D. Candidate, Johns Hopkins University. Email: achatto1 at jhu dot edu dot com
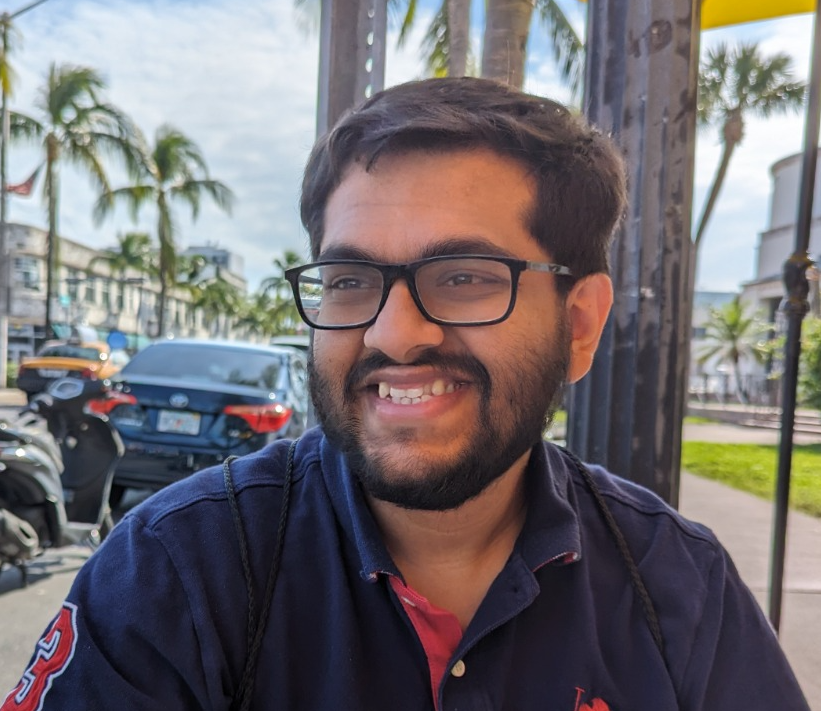
Office: 322, Clark Hall, JHU
Baltimore, MD, 21218
About me
I am currently a Ph.D. candidate in the Computer Science Department at the Johns Hopkins University (JHU). My Ph.D. advisors are Prof. René Vidal and Prof. Donald Geman.
Ph.D. dissertation topic: An Information-theoretic Framework for Designing Interpretable Predictors.
Before JHU, I did my M.S. in Computational Natural Sciences and B.Tech in Computer Science from the International Institute of Information Technology, Hyderabd (IIIT-H). My M.S. advisor was Prof. U. Deva Priyakumar.
M.S. dissertation topic: A probabilistic framework for constructing temporal relations in Monte Carlo trajectories.
Research Interests
My current research involves developing Machine Learning (ML) algorithms that not only make accurate predictions but also provide human-interpretable explanations for their predictions (the users of these algorithms may not be ML experts themselves). Given that ML algorithms are increasingly being deployed in critical decision-making areas such as finance and healthcare, ensuring the interpretability of these algorithms is essential. For more information about my work, please visit the Projects section.
Apart from developing ML algorithms, I am also keenly interested in their application in biology. In the past, I worked on developing ML techniques to understand the unfolding of biomolecules (proteins + RNAs) from their molecular dynamic trajectories. To know more about this work, visit the Projects section.
News
Jan 15, 2024 | Our paper on an interpretable-by-design framework for image classification using Large Language and Vision models integrated with Information Pursuit (a greedy algorithm that makes predictions by sequentially asking informative questions about the input image) accepted as a conference paper at ICLR 2024! |
---|---|
Sep 22, 2023 | Our paper on an information theoretic perspective of Orthogonal Matching Pursuit and its applications to explainable AI was accepted at NeurIPS 2023 as a spotlight presentation! (acceptance rate: 3%). |
Sep 16, 2023 | Presenting a poster at DeepMath 2023. |
Jan 21, 2023 | Our paper on an information theoretic framework for making interpretable predictions (explainable-AI) was accepted as a journal paper at TPAMI, with a follow-up more efficient version accepted as a conference paper at ICLR 2023! |
Sep 18, 2022 | Presenting a poster at DeepMath 2022. |
May 6, 2021 | Honoured to receive the MINDS Fellowship for Spring and Summer semesters in 2021. |
Sep 9, 2019 | Our work on explainable-AI advocating using causality to interpret neural networks via post-hoc attribution has been featured by Hindustan Times. Check out the article here ![]() ![]() |
Selected Publications
- ICLRBootstrapping Variational Information Pursuit with Large Language and Vision Models for Interpretable Image ClassificationIn The Twelfth International Conference on Learning Representations, 2024